class: center, middle, inverse, title-slide # Econ 474 - Econometrics of Policy Evaluation ## Instrumental Variables ### Marcelino Guerra ### February 16-23, 2021 --- # IV and Causality I The most important contemporary use of IV methods is to solve the problem of missing or unknown control variables - the omitted variable bias (OVB). Let's recall the discussion about short vs. long regression using earnings and schooling. The regression we seek to estimate is: `$$\underbrace{Y_{i}}_{Wages}=\alpha+\rho \underbrace{s_{i}}_{Schooling}+\gamma \underbrace{A_{i}}_{ability}+v_{i}$$` Assume that all we need to estimate the causal effect of education on wages is schooling and ability. Hence, `\(E(s_{i}v_{i})=0\)`, i.e., the error term is uncorrelated with schooling and the regression of `\(Y_{i}\)` on `\(s_{i}\)` and `\(A_{i}\)` will give the coefficients we seek. -- Say we don't observe the individuals' ability. Hence, the regression we have is `\(Y_{i}=\alpha+\rho s_{i}+\eta_{i}\)`, where `\(\eta_{i}=\gamma A_{i}+v_{i}\)`. Now, the residuals of the regression you can estimate are correlated with `\(s_{i}\)`, and `$$\frac{Cov(Y_{i},s_{i})}{V(s_{i})} \neq \rho$$` -- What you have running the short regression is `\(\rho^{s}=\rho^{l}+bias\)`. **What IV does is to recover the long regression without directly observing `\(A_{i}\)`.** --- # IV and Causality II Potential earnings can be written as `$$\small Y_{0i}=\alpha+\eta_{i}=\alpha+\gamma A_{i}+v_{i}$$` and, as mentioned before, we are happy to assume `\(E(s_{i}v_{i})=0\)`. The causal effect of schooling is `\(Y_{1i}-Y_{0i}=\rho\)`. More precisely, move from `\(s-1\)` to `\(s\)` years of schooling and assuming constant returns to education: `$$\small Y_{si}-Y_{(s-1)i}=\rho$$` -- Can we recover the true causal effect without observing `\(A_{i}\)`? That's possible with a **valid instrument**. A valid instrument `\(Z_{i}\)` is correlated with the causal variable of interest `\(s_{i}\)` and uncorrelated with any other determinants of the dependent variable. In other words, `\(Z_{i}\)` has to be 1. Correlated with the treatment `\(s_{i}\)`, i.e., `\(C(z_{i}, s_{i}) \neq 0\)` a.k.a **inclusion restriction** 2. Uncorrelated with `\(\eta_{i}=\gamma A_{i}+v_{i}\)` and hence with `\(Y_{0i}\)` `\((C(z_{i}, \eta_{i}) = 0)\)` -- `\(z_{i}\)` does not influence `\(Y_{i}\)` except through `\(s_{i}\)` - a.k.a **exclusion restriction** `\((E[Z_{i}\eta_{i}]=0)\)`. From the short regression and using the exclusion restriction: `$$\small\rho=\frac{Cov(Y_{i},Z_{i})}{Cov(s_{i}, Z_{i})}=\frac{Cov(Y_{i},Z_{i})/V(Z_{i})}{Cov(s_{i}, Z_{i})/V(Z_{i})}=\frac{\text{Reduced Form}}{\text{First Stage}}$$` --- # Wald Estimator Back to the long regression: `$$\underbrace{Y_{i}}_{Wages}=\alpha+\rho \underbrace{s_{i}}_{Schooling}+\gamma \underbrace{A_{i}}_{ability}+v_{i}=\alpha+\rho s_{i}+\eta_{i}$$` Assuming the instrument `\(Z_{i}\)` is itself a **dummy variable**, `$$\frac{Cov(Y_{i}, Z_{i})}{V(Z_{i})}=E[Y_{i}|Z_{i}=1]-E[Y_{i}|Z_{i}=0]$$` -- and an analogous formula applies to `\(\frac{Cov(s_{i}, Z_{i})}{V(Z_{i})}\)`. Hence `$$\rho=\frac{E[Y_{i}|Z_{i}=1]-E[Y_{i}|Z_{i}=0]}{E[s_{i}|Z_{i}=1]-E[s_{i}|Z_{i}=0]}$$` -- The simplest IV construct is composed by two differences in means: the **Wald estimator**. You can get the same result using `\(E[\eta_{i}|z_{i}]=0\)` on the short regression. --- class: inverse, middle, center # Does Compulsory School Attendance Affect Schooling and Earnings? --- # Example: Earnings and Compulsoring Schooling I * Children born in late-quarters start school younger - in most states, children enter kindergarten in the year they turn 5, whether or not they've had a fifth birthday by the time school starts * US compulsory schooling laws are **in terms of age**, not number of years completed at school. Usually, states allow students to leave school at 16 whether or not they've finished the school year -- * The combination of once a year entrance and cutoffs based on birthdays generate variation in years of education for students who drop out as soon as they can. * Since there is a good deal of randomness to dates of birth, that mimics an experimental random assignment. Angrist and Krueger (1991)<sup>1</sup> explored that scenario using quarter of birth as IV. * Late quarter of births (QOB) have more years of schooling (high school, not college) * The authors estimate the returns to compulsory schooling comparing average schooling and earnings for early and late-quarter births .footnote[Joshua D. Angrist and Alan B. Krueger, "Does Compulsory School Attendance Affect Schooling and Earnings?" *Quarterly Journal of Economics*, November 1991.] --- # Example: Earnings and Compulsoring Schooling II .pull-left[ <img src="lec4_files/figure-html/unnamed-chunk-1-1.png" width="900px" style="display: block; margin: auto;" /> .small[**Note: This figure plots average schooling by quarter of birth (QOB) for men born in 1930-1939 in the 1980 U.S Census. Angrist and Krueger (1991).**] ] .pull-right[ <img src="lec4_files/figure-html/unnamed-chunk-2-1.png" width="900px" style="display: block; margin: auto;" /> .small[**Note: This figure plots average log weekly wages by quarter of birth (QOB) for men born in 1930-1939 in the 1980 U.S. Census. Angrist and Krueger (1991).**] ] --- # Earnings and Compulsoring Schooling III .pull-left[A simple QOB-based IV compares the schooling and earnings of men born in the fourth quarter to the schooling and earnings of men born in earlier quarters `$$\text{Effect of schooling on wages}=\\ \frac{\text{Effect of QOB on wages}}{\text{Effect of QOB on schooling}}=\\ \frac{\text{Reduced Form}}{\text{First Stage}}$$` For Men born in 1930-1930, the returns to schooling are `\(\frac{-0.0111}{-0.1088}=0.102\)`, i.e., 10.2% increase in wages. That is higher than the OLS return to education estimates (around 7.1%). ] .pull-right[ 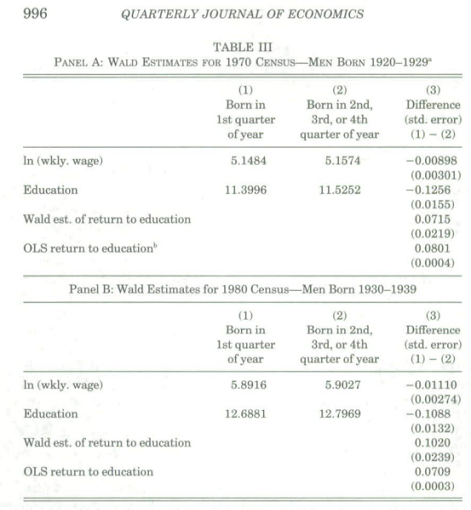 ] --- # Two-Stage Least Squares (2SLS) I We take advantage of IV by doing 2SLS. 2SLS allows you to include more than one instrument (not necessarily a good idea) and also other covariates in the first-stage and reduced form equations `$$Y_{i}=\alpha^{'}X_{i}+\rho s_{i}+\eta_{i} \text{ } (1)$$` The first stage and reduced form equations are `$$s_{i}=X_{i}^{'}\pi_{10}+\pi_{11}^{'}Z_{i}+\varepsilon_{1i}=\hat{s_{i}}+\varepsilon_{1i} \text{ } (2)$$` `$$Y_{i}=X_{i}^{'}\pi_{20}+\pi_{21}^{'}Z_{i}+\varepsilon_{2i} \text{ } (3)$$` -- The 2SLS second-stage is `$$Y_{i}=\alpha^{'}X_{i}+\rho \hat{s_{i}}+\varepsilon_{2i} \text{ } (4)$$` -- You get equation (4) substituting (2) on (1). `\(\hat{s}_{i}\)` is a linear combination of covariates `\(X\)` and the instrument `\(Z\)`, and that is not correlated with the error term of the structural equation (1). Hence, the OLS estimation of (4) identifies `\(\rho\)`. In practice, we don't run those regressions manually. **We let
do it for us!** --- # Two-Stage Least Squares (2SLS) II Applying the same reasoning, we get the reduced-form: `$$Y_{i}=\alpha^{'}X_{i}+\rho[X_{i}^{'}\pi_{10}+\pi_{11}^{'}Z_{i}]+\rho \varepsilon_{1i} +\eta_{i} \text{ } (5)$$` `$$=X_{i}^{'}[\alpha+\rho\pi_{10}]+\rho\pi_{11}^{'}Z_{i}+[\rho \varepsilon_{1i}+\eta_{i}]$$` `$$=X_{i}^{'}\pi_{20}+\pi_{21}^{'}Z_{i}+\varepsilon_{2i}$$` The 2SLS implicitly computes the ratio `\(\frac{\text{Reduced-form}}{\text{First-Stage}}\)`: `$$\frac{\pi_{21}}{\pi_{11}}=\rho$$` --- # 2SLS Results I .pull-left[ * The table compares the OLS with the 2SLS estimates for different specifications that add controls and use multiple instruments. Notice that **if you are adding covariates, they should be included in both the first and second stages** * 2SLS estimates in this case are larger than OLS estimates and robust to the inclusion of covariates The requirements for the IV strategy to capture the causal effect are: 1. Instruments must predict the treatment variable (**strong first stage/F-stat > 10**) 2. Instruments should be as good as random (should be independent of omitted variables) 3. Instruments should affect the outcome solely through the variable to be instrumented ] .pull-right[ 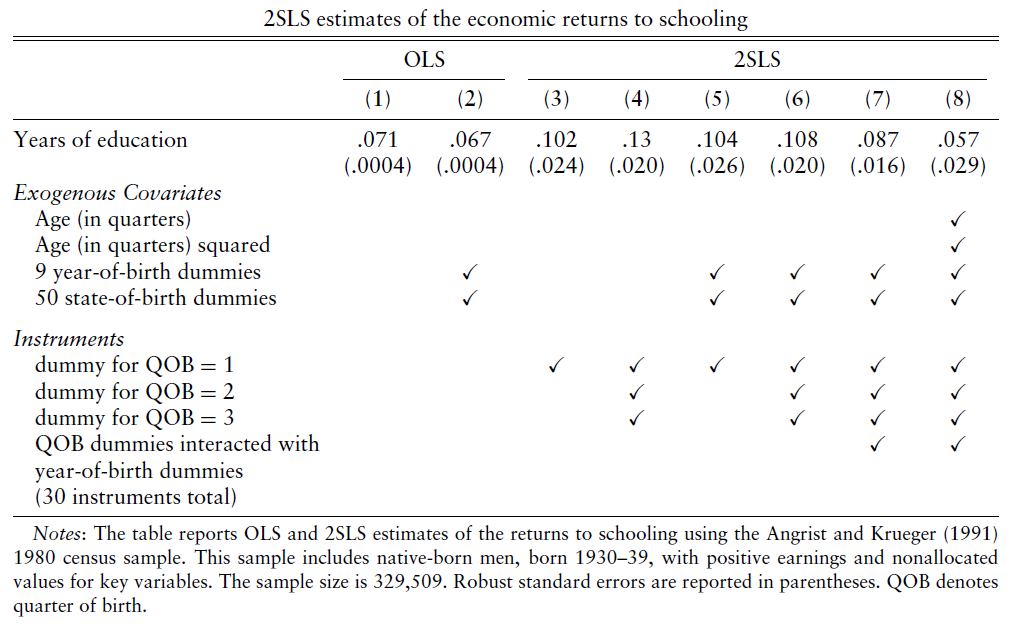 .small[**Results for men born in 1930-1939 in the 1980 U.S. Census. Source: Angrist and Krueger (1991).**] ] --- # 2SLS Results II .pull-left[ * **Is QOB independent of other characteristics?** * What if QOB is related to season flu, which affects babies? (i.e., is it bad to be born during the flu season?) * Studies found maternal schooling peaks for mothers who gave birth in the second quarter. On the other hand, the seasonal pattern in schooling and wages shows peaks in the third and fourth quarters, and family background cannot account for that * **What if school-starting age matters itself?** * **Internal *vs.* External validity** * The study has no say on returns of college education * What if men dropping out of high school is a special kind of population? ] .pull-right[ 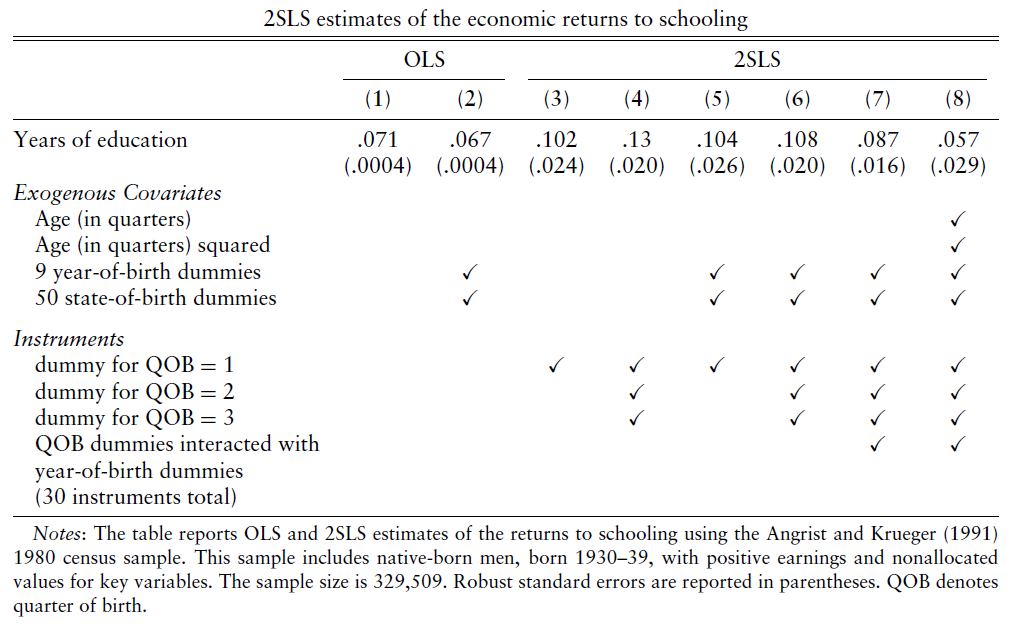 .small[**Results for men born in 1930-1939 in the 1980 U.S. Census. Source: Angrist and Krueger (1991).**] ] --- class: inverse, middle, center # Children and Their Parents' Labor Supply: Evidence from Exogenous Variation in Family Size --- # Example: Children and their Parents Labor Supply I * Economists and demographers have developed models linking family and labor market, and empirical studies of childbearing and labor supply are designed to test those models * For instance, the link between fertility and labor supply might partially explain the postwar increase in women's labor-force participation rates * Other interesting links are the impact of reductions in female labor supply on total time parents devote to child care, wives' earnings effect on marital stability, among others -- * Although most of the studies suggest a negative correlation between fertility and female labor supply, the empirical analysis is complicated by the fact that **fertility and labor supply are jointly determined** -- * Agrist and Evans (1996)<sup>1</sup> tackle this endogeneity using an instrumental variable (IV) based on the sibling sex mix in families with two or more children and also twining at the second birth. .footnote[Joshua D. Angrist and William N. Evans, "Children and Their Parents' Labor Supply: Evidence from Exogenous Variation in Family Size" *The American Economic Review*, 1996.] --- # Example: Children and their Parents Labor Supply II * Because sex mix is as good as random, a dummy for whether the sex of the second child matches the sex of the first child is a plausible valid instrument for further childbearing among women with at least two children * Parents of same-sex siblings are substantially more likely to go on to have a third child * Twining at the second birth is also used as an instrument to identify the impact of moving from the second to the third child -- .center[**Those two instruments are part of the "fertility quasi-experiment"**] -- * The question is: how a third child affects maternal labor supply? One can write the causal model for the impact of a third child on a mother's participation in the labor market as `$$Y_{i}=Y_{0i}+D_{i}(Y_{1i}-Y_{0i})=\alpha+\rho D_{i}+\eta_{i}$$` -- * Data comprehends (mostly) married women with at least two children. The dependent variables are **employment**, **hours worked**, **weeks worked**, and **earnings**. The treatment variable `\(D_{i}\)` is equal to one if mothers have more than two kids. The instrument `\(Z_{i}\)` indicates whether there were multiple second births (twins) or same-sex siblings at second birth --- # Example: Children and their Parents Labor Supply III .pull-left[ With a binary instrument, the IV estimand is the Wald formula: `$$\small\rho=\frac{Cov(Y_{i},Z_{i})}{Cov(D_{i}, Z_{i})}=\frac{E[Y_{i}|Z_{i}=1]-E[Y_{i}|Z_{i}=0]}{E[D_{i}|Z_{i}=1]-E[D_{i}|Z_{i}=0]}$$` The twins' first stage is shown in column 8. Since `\(D_{i}\)` is a dummy, the first stage represents a probability - 0.6031. To interpret that, think about the other way around, i.e., 1-0.6=0.4. 40% represents the probability of a woman who doesn't have twins in the second birth having a third child. The fact that twins come at the second birth transforms that probability into a certainty. The first stage of the same sex is 0.06. The underlying probabilities are 37% and 43%: mothers with mixed-sex siblings have a 37% probability of having a third child. The fact that the mother has same-sex siblings adds 6% to that. ] .pull-right[ 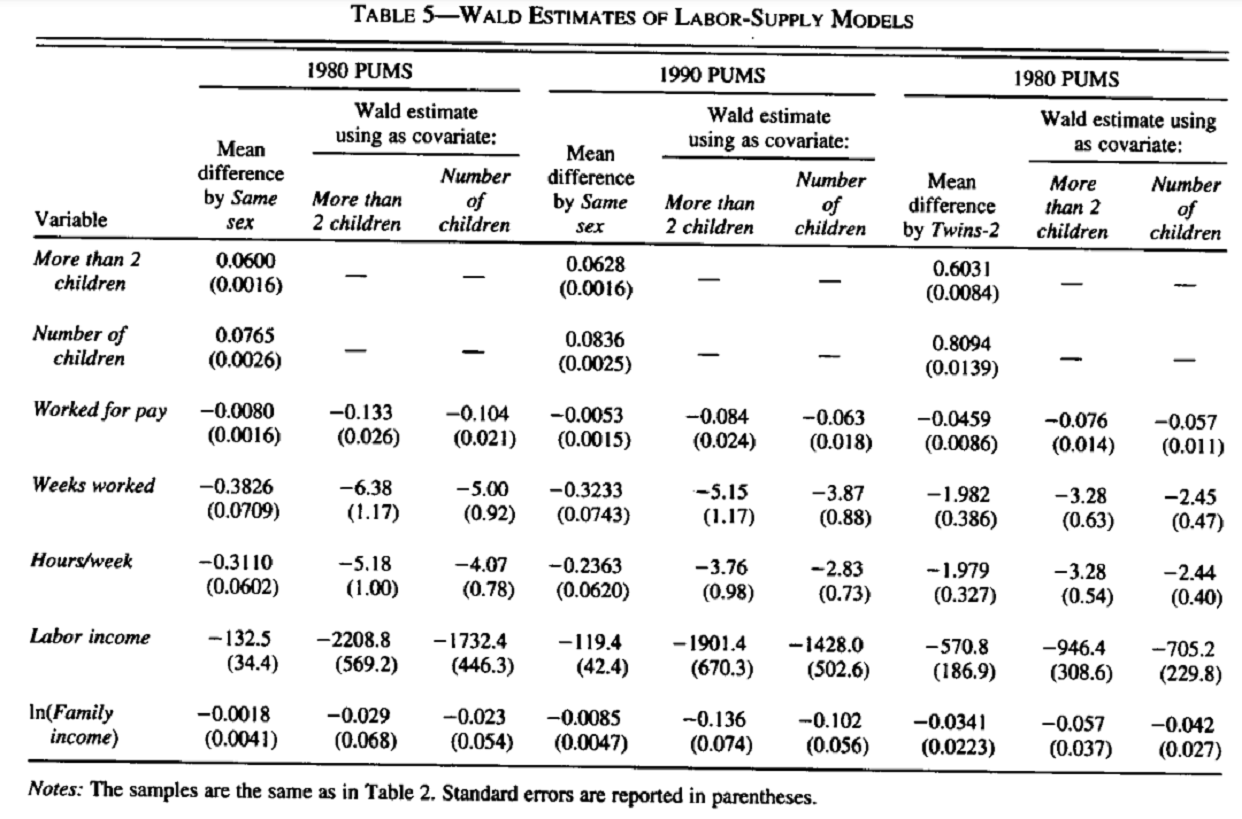 .small[**Source: Angrist and Evans (1996).**] ] --- # Example: Children and their Parents Labor Supply IV .pull-left[ The reduced forms of interest are shown in columns 2 and 8. * Mothers with same-sex siblings (column 2) have less participation in the labor market (about 1% less), work less (about 1/3 of an hour), and earn $132.5 less compared to mothers with one boy-one girl. Apply the same reasoning to column 8, and you get the reduced-form using twinning (which has much bigger values) * Dividing the reduced-form by the first stage, the IV estimates show the causal effect of a third child on labor supply * The participation in the labor market drops by 7.6-13.3 points * Weeks worked drop by 3.28-6.38 * Hours per week drop by 3.28-5.18 * Earnings from labor are also lower: $946.4-2,208.8 ] .pull-right[ 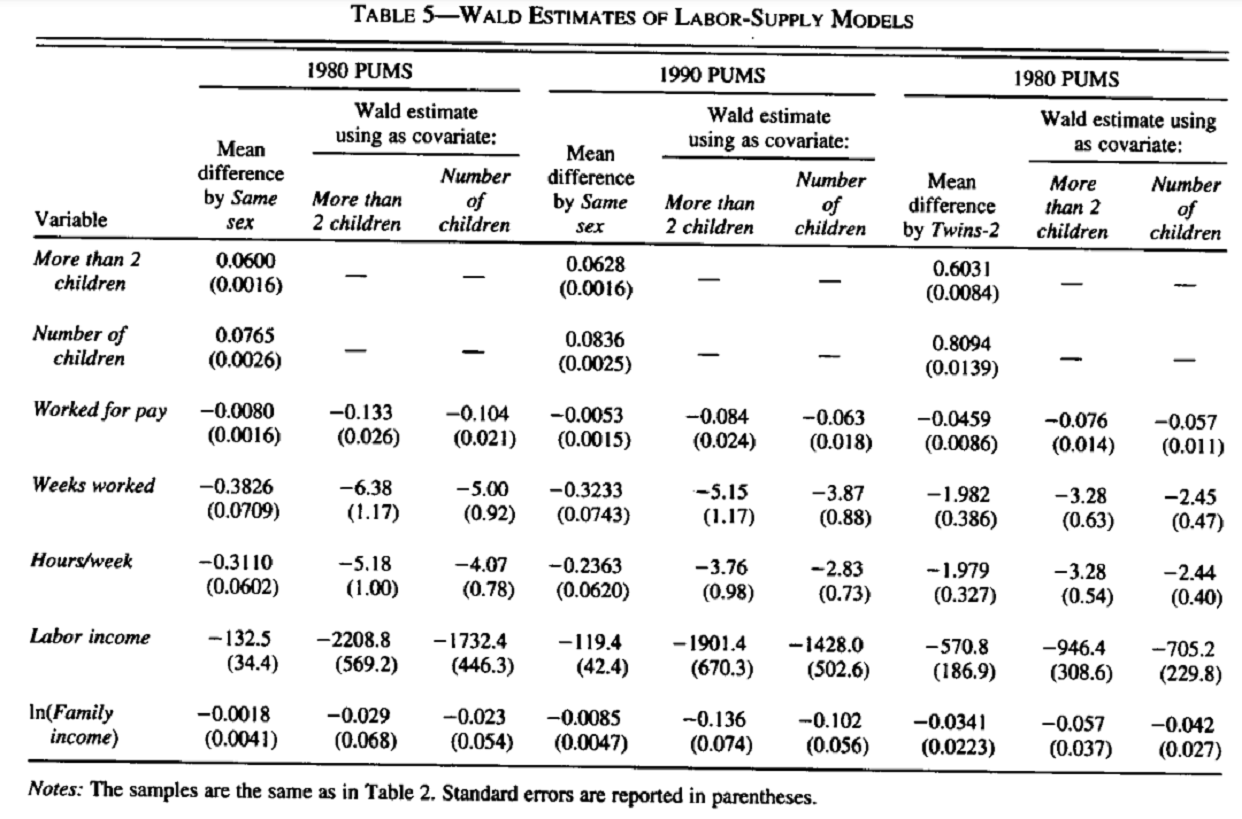 .small[**Source: Angrist and Evans (1996).**] **The effects generated by using distinct IVs (assuming both are valid) are entirely different in magnitude. Why are the IV estimates instrumenting by twins smaller? What does drive the heterogeneity?** ] --- class: inverse, middle, center # IV in Randomized Trials --- # KIPP Charter School I .pull-left[ * Charter schools are public-funded schools that operate with more autonomy than traditional American public schools - they are free to structure their curricula and school environments. Many charter schools have longer school days and activities during weekends and summer * Another particular characteristic of Charter schools is their teachers and staff rarely belong to labor unions, unlike public sector teachers * The documentary **[Waiting for Superman](https://www.youtube.com/watch?v=yFN0nf6Hqk0)** features Charter schools and argues that these schools offer the best hope for poor minority students ] .pull-right[ 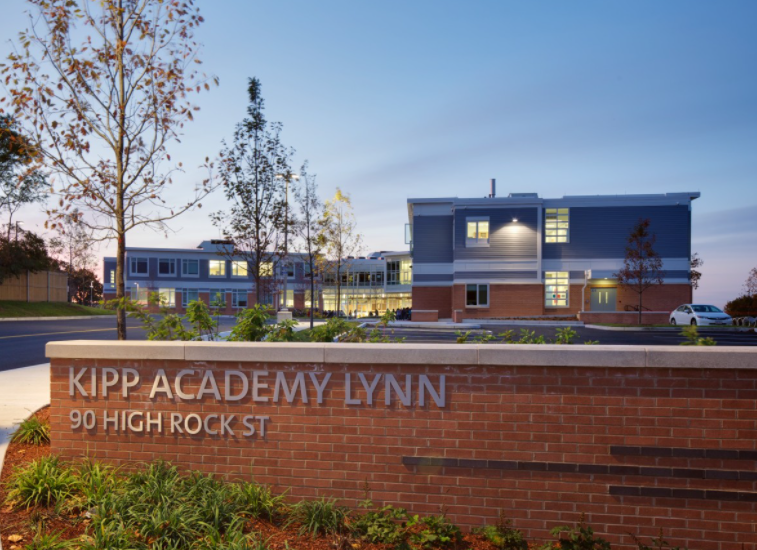 .small[**KIPP Middle School in Lynn, Massachusetts.**]] --- # KIPP Charter School II .pull-left[ * Many Charter schools are affiliated with the Knowledge Is Power Program (KIPP). KIPP schools follow a model that emphasizes discipline and comportment, selective teacher hiring, and focus on traditional reading and math skills * The KIPP network serves a student body that is 95% black and Hispanic, with most students eligible for federal subsidies * Charter students do better than their public school peers: **is there a causal effect?** * The American debate over education reform focuses on the achievement gap. Because of its focus on minorities, KIPP is often central in this debate. KIPP skeptics argue that KIPP's success reflects the fact those schools attract families whose children are more likely to do better anyway ] .pull-right[ 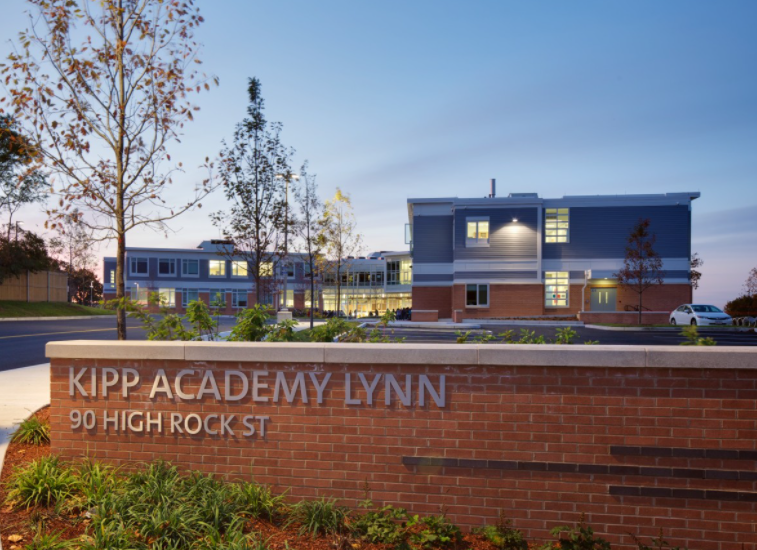 .small[**KIPP Middle School in Lynn, Massachusetts.**]] --- # Playing lottery I .pull-left[ * KIPP in Lynn-MA opened in Fall 2004. After 2005, the demand for seats accelerated, with more than 200 applicants for about 90 seats in fifth grade each year. **Since Massachusetts requires scarce seats to be allocated by lottery**, seats were offered by chance * Although the decision to attend a charter school is never entirely random, comparisons of applicants who are and are not **offered** a seat as a result of a lottery should be apples to apples in nature. Assuming that winning the lottery affects grades only through increasing the probability of attending the charter school, **IV turns randomized offers into causal estimates of the effect of charter attendance** ] .pull-right[ 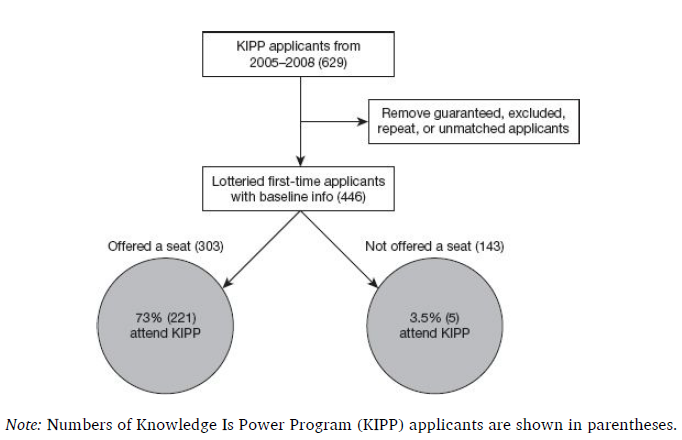 .small[**Figure 3.1, Angrist and Pischke (2014).**]] --- # Playing lottery II .pull-left[ * The table summarizes important information about KIPP applicants' baseline characteristics (Panel A) and outcomes (Panel B). As a benchmark, column 1 displays information about all Lynn public school fifth graders * The comparison between lottery winners and losers is indeed apples to apples: they are equally likely to be black or Hispanic, eligible for free lunch, and also had similar performance in tests during the fourth grade * Column 4 reports information about students who enrolled at KIPP Lynn, and column 5 compares **KIPP applicants who did and did not enroll at KIPP** * We can see that the offer of a seat at KIPP Lynn boosts math scores by `\(.36 \sigma\)` (Panel B, column 3). What does that tell us about **the effect of KIPP Lynn attendance?** ] .pull-right[ 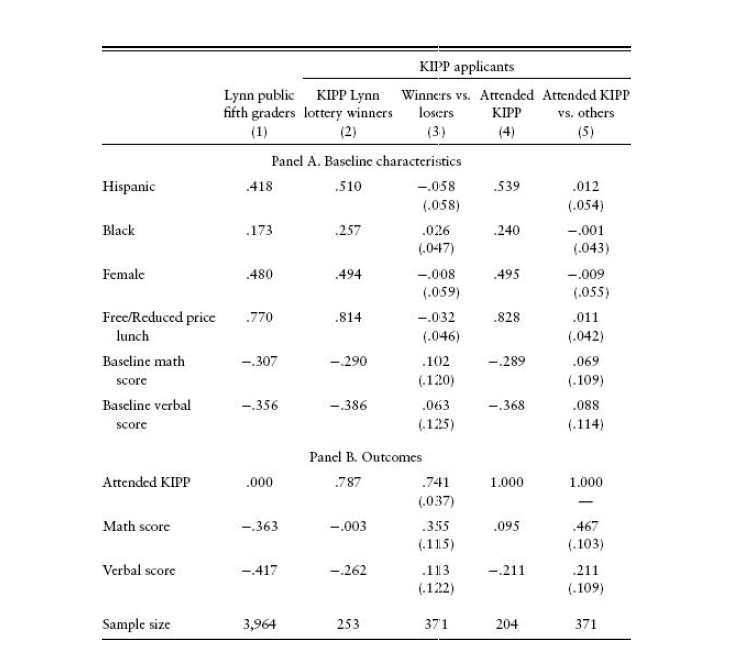 .center[.small[**Table 3.1, Angrist and Pischke (2014).**]]] --- # IV Results .pull-left[ The instrument `\(Z_{i}\)` is a dummy variable that takes on 1 if the student received an offer. The treatment variable `\(D_{i}\)` is also a dummy that identifies students who attended KIPP Lynn. Applying the Wald formula: `$$\small\rho=\frac{Cov(Y_{i},Z_{i})}{Cov(D_{i}, Z_{i})}=\frac{E[Y_{i}|Z_{i}=1]-E[Y_{i}|Z_{i}=0]}{E[D_{i}|Z_{i}=1]-E[D_{i}|Z_{i}=0]}$$` `$$\rho=\frac{-0.003-(-0.358)}{0.787-0.046}=0.48 \sigma$$` The logic behind the formula is that KIPP offers are assumed to affect test scores via KIPP attendance alone, and those offers increase attendance rates by around 75%. Adjusting the effect of offers on scores by that proportion, you have **the causal impact of attendance on test scores**. ] .pull-right[ 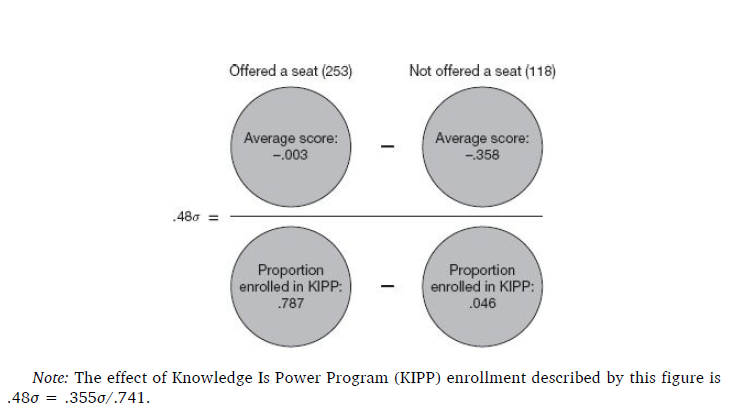 .small[**Figure 3.2, Angrist and Pischke (2014).**]] --- class: inverse, middle, center # Local Average Treatment Effects (LATE) --- # LATE framework In the IV setting, the engine that drives the causal effect is the instrument `\(Z_{i}\)`, but the variable of interest is still `\(D_{i}\)`. This IV feature leads us to adopt a generalized version of the potential outcome notation. Denote the potential outcome for `\(i\)` as `\(Y_{i}(d,z)\)`, where `\(D_{i}=d\)` and `\(Z_{i}=z\)`. We can think of IV as initiating a causal chain where `\(Z \rightarrow D \rightarrow Y\)`. -- To build these links, let's define **potential treatment status** indexing it by values of `\(Z_{i}\)` * `\(D_{1i}\)` is i's treatment status when `\(Z_{i}=1\)` * `\(D_{0i}\)` is i's treatment status when `\(Z_{i}=0\)` * We only observe one of these, and the **observed treatment status** is `\(D_{i}=D_{0i}+(D_{1i}-D_{0i})Z_{i}\)` * The causal effect of `\(Z_{i}\)` on `\(D_{i}\)` is `\(D_{1i}-D_{0i}\)` (**first stage**) -- **LATE Assumptions** 1. Independence 2. Exclusion Restriction 3. Strong first-stage 4. Monotonicity --- # LATE assumptions **Independence `\(\neq\)` Exclusion** **Independence** means that the instrument is as good as randomly assigned and implies that the first stage is the average causal effect of `\(Z_{i}\)` on `\(D_{i}\)` -- **Exclusion restriction** is the assumption that the instrument `\(Z_{i}\)` only affects `\(Y_{i}\)` through `\(D_{i}\)`. Using the exclusion restriction, we can define potential outcomes indexed solely against treatment status `$$Y_{i}(1,1)=Y_{i}(1,0)\equiv Y_{1i}$$` `$$Y_{i}(0,1)=Y_{i}(0,0)\equiv Y_{0i}$$` and the observed outcome can be written as `$$Y_{i}=Y_{i}(0, Z_{i})+[Y_{i}(1, Z_{i})-Y_{i}(0, Z_{i})]D_{i}=\\Y_{0i}+(Y_{1i}-Y_{0i})D_{i}$$` -- The **monotonicity** assumption states that either `\(D_{1i} \geq D_{0i}\)` or `\(D_{1i} \leq D_{0i}\)` for everyone. In other words, **while the instrument may not affect some people, all those affected are affected in the same way** - the instrument either increases or decreases the chances of being treated for everyone. --- # LATE compliers Given the independence, exclusion restriction, strong first stage and monotonicity, we have the **LATE Theorem**: `$$\frac{E[Y_{i}|Z_{i}=1]-E[Y_{i}|Z_{i}=0]}{E[D_{i}|Z_{i}=1]-E[D_{i}|Z_{i}=0]}=E[Y_{1i}-Y_{0i}|D_{1i}>D_{0i}]$$` which does not change what we have been doing so far (Wald formula), but improves our understanding of the results we are getting: LATE is the average causal effect of `\(D_{i}\)` on `\(Y_{i}\)` for those whose treatment status is manipulated by the instrument. -- The LATE framework partitions any population with an instrument into a set of three instrument-dependent subgroups: *Compliers*: LATE compliers have `\(D_{1i}>D_{0i}\)` *Always-takers*: `\(D_{1i}=D_{0i}=1\)` *Never-takers*: `\(D_{1i}=D_{0i}=0\)` IV is only informative about **compliers**. --- # KIPP Charter School Example .pull-left[ * There are four types of children who applied to KIPP in Lynn. Applicants like *Camila* are happy to enroll at KIPP if they win a seat but would accept to go elsewhere if they lose the lottery (**compliers**). Applicants like *Normando* would never attend KIPP, even winning the lottery (**never-takers**). Finally, applicants like *Alvaro* would find a way to enroll at KIPP even when they lose the lottery - these are **always-takers**. * Camila is the type of applicant that gives power to IV because the instrument changes her treatment status, and LATE is the average causal effect of KIPP attendance on **compliers** (like Camila) who enroll at KIPP if and only if they win the lottery ] .pull-right[ 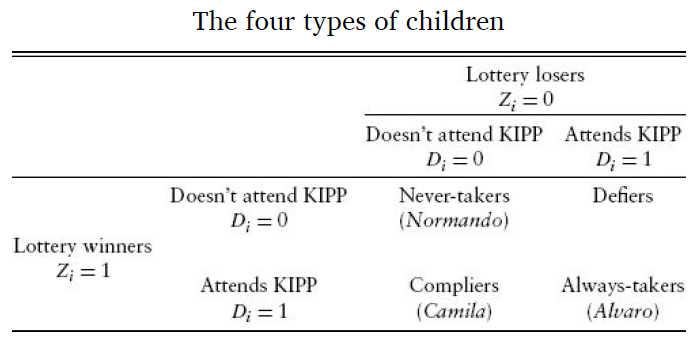 .small[**Table 3.2, Angrist and Pischke (2014).**] The presence of **defiers** - those who enroll at KIPP only when not offered a seat in the lottery - makes IV hard to interpret. Defiers are unlikely in lotteries and other IV settings, and the monotonicity assumption rules out their presence: your instrument should affect treatment status in only one direction. ] --- # LATE and TOT Sometimes, we are interested in average causal effects for the entire treated population: the **treatment effect on the treated (TOT)**: `$$E[Y_{1i}-Y_{0i}|D_{i}=1]$$` In this framework, there are two ways to be treated - i.e., to have `\(D_{i}\)` switched on. One is to be treated regardless the instrument is switched off or on (like Alvaro, the **always-takers**). The rest of the treated population is represented by the **compliers** who were randomly assigned `\(Z_{i}=1\)`, like Camila. -- Because the treated population includes always-takers, LATE and TOT are usually not the same. Also, the average causal effect of Charter school attendance might be different in other settings (e.g., charter schools with fewer minority applicants). One needs to assess the **external validity** of those LATE findings.